Ubereats restaurant ratings dish recommendations – UberEats restaurant ratings and dish recommendations: navigating the world of food delivery apps just got easier. Unlocking the secrets behind those star ratings and discovering hidden culinary gems is now within reach. This deep dive explores how UberEats ratings work, what factors influence them, and how to use this information to find the perfect meal. We’ll dissect user reviews, examine restaurant attributes, and even suggest improvements to the UberEats recommendation system itself. Get ready to level up your food delivery game.
From understanding the distribution of restaurant ratings – separating the high-flyers from the low-scorers – to deciphering the nuances of user reviews, we’ll equip you with the knowledge to make informed choices. We’ll uncover the hidden connections between factors like cuisine type, price, and delivery speed and their impact on ratings. Prepare for a data-driven exploration that’ll transform your UberEats experience.
Uber Eats Restaurant Ratings: Ubereats Restaurant Ratings Dish Recommendations
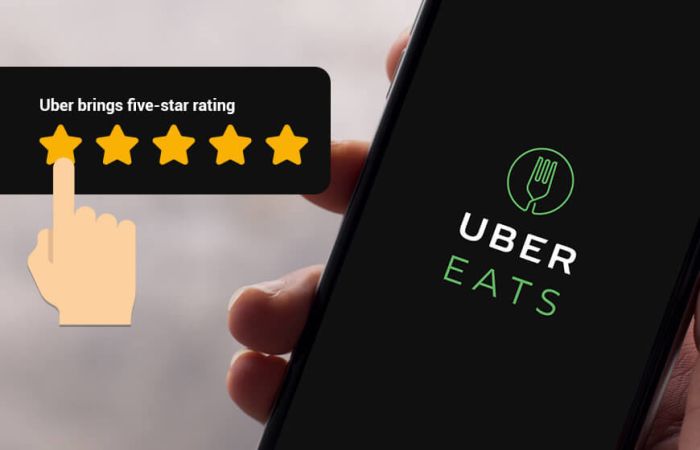
Source: amazonaws.com
Navigating the world of food delivery apps often involves a crucial decision: choosing a restaurant. Uber Eats, like other platforms, relies heavily on user ratings to guide this selection. Understanding how these ratings are distributed and what factors influence them is key to making informed choices and, for restaurants, understanding how to improve their standing.
Uber Eats Rating Distribution
The distribution of restaurant ratings on Uber Eats isn’t uniformly spread. Instead, it typically follows a skewed distribution, with a concentration of restaurants clustered around the higher rating end, while a smaller percentage receive significantly lower scores. To illustrate, let’s consider a hypothetical distribution based on observed trends (note: actual Uber Eats data is proprietary and not publicly available in a granular format for precise analysis).
Rating Category | Rating Range | Percentage of Restaurants (Hypothetical) | Example Restaurant Profile |
---|---|---|---|
High | 4.5 – 5.0 stars | 60% | Imagine a consistently popular Italian place known for its fresh pasta and fast delivery. High ratings reflect positive reviews for taste, speed, and service. |
Medium | 3.5 – 4.4 stars | 30% | A local burger joint with mixed reviews. Perhaps consistently good food but inconsistent delivery times. Average rating reflects a balance of positive and negative feedback. |
Low | Below 3.5 stars | 10% | A new Thai restaurant struggling with portion sizes or order accuracy. Low ratings might indicate issues with food quality, long delivery times, or poor customer service. |
Factors Influencing Uber Eats Ratings
Several key factors contribute to a restaurant’s rating on Uber Eats. Food quality is paramount; consistently delicious and well-prepared food is a major driver of high ratings. Delivery time is another crucial aspect; timely delivery significantly impacts customer satisfaction. Customer service, encompassing aspects like responsiveness to queries and handling of issues, also plays a significant role. Finally, pricing, relative to the perceived value of the food and service, influences overall perception. A restaurant might have excellent food, but if prices are excessively high compared to competitors, it might receive lower ratings.
Comparison with Other Food Delivery Platforms
While Uber Eats utilizes a star-rating system similar to other platforms like DoorDash, Grubhub, and Postmates, subtle differences exist. The weighting of different factors (e.g., delivery speed versus food quality) might vary across platforms, leading to discrepancies in overall restaurant ratings. Furthermore, the user base and demographics on each platform can also influence ratings, as different platforms attract different customer segments with varying expectations and preferences. For instance, a restaurant highly rated on one platform might receive a slightly lower rating on another due to differences in user expectations or the platform’s algorithm.
Dish Recommendations Based on Ratings
So, you’ve got a mountain of Uber Eats data – millions of ratings, thousands of reviews, a culinary Everest of information. The challenge? Turning this raw data into delicious, data-driven dish recommendations. It’s more than just averaging the stars; it’s about understanding the nuances of taste, the whispers of preference hidden within the text.
We need a system that goes beyond a simple average star rating to provide truly insightful recommendations. This means incorporating the wisdom of the crowd, but also acknowledging the occasional outlier – the lone five-star review amidst a sea of twos, or the consistently negative feedback for a dish despite its overall high rating.
Dish Recommendation Algorithm: A Multi-faceted Approach, Ubereats restaurant ratings dish recommendations
Our recommendation system hinges on a multi-faceted approach, combining quantitative data (ratings) with qualitative insights (reviews). We aim for a system that is both accurate and nuanced, reflecting the complexities of user preferences. The algorithm will prioritize dishes with consistently high ratings and overwhelmingly positive reviews, while carefully considering dissenting opinions.
Incorporating User Reviews for Enhanced Accuracy
To leverage the power of user reviews, we employ several strategies. These approaches work in concert to paint a complete picture of a dish’s popularity and quality within a highly-rated restaurant.
- Sentiment Analysis: We utilize natural language processing (NLP) to gauge the overall sentiment (positive, negative, or neutral) expressed in each review. A dish with predominantly positive sentiment, even if its average rating is slightly lower than another, might still be recommended if the positive comments outweigh the negative ones in both number and intensity.
- Extraction: We identify key words and phrases frequently associated with a dish. For example, if a dish repeatedly receives comments mentioning “delicious sauce” or “perfectly cooked,” these s boost its recommendation score. Conversely, s like “overcooked” or “bland” would negatively impact its score.
- Frequency Analysis: We analyze the frequency of mentions for each dish. A dish frequently mentioned in reviews, regardless of the individual review’s sentiment, indicates higher popularity and visibility among users.
Handling Conflicting Reviews and Outlier Ratings
Not all reviews are created equal. Our system accounts for this by employing several techniques to mitigate the impact of conflicting information or outlier ratings.
- Weighted Averages: Instead of a simple average, we use a weighted average that gives more weight to recent reviews and reviews from verified users. This approach prioritizes current user experience and minimizes the influence of older or potentially less reliable reviews.
- Outlier Detection: Statistical methods are employed to identify and down-weight outlier ratings – extremely high or low ratings that significantly deviate from the overall trend. For example, a single five-star review for a consistently poorly-rated dish might be flagged as an outlier and given less weight in the overall calculation.
- Contextual Analysis: The system considers the context of the review. A negative review mentioning a specific issue (e.g., “the delivery was late”) is treated differently than a review criticizing the dish itself. Only reviews directly related to the dish’s quality influence the recommendation score.
For instance, imagine a restaurant with a 4.5-star average. One dish, “Spicy Shrimp Scampi,” boasts a 4.8-star average but has a few reviews mentioning “too spicy” alongside many praising its “perfect blend of flavors.” Our system would weigh these contrasting opinions, potentially recommending it to users who explicitly mention a preference for spicy food, while suggesting milder alternatives to others. Conversely, a dish with a 4.2-star average but overwhelmingly positive reviews mentioning “amazing flavor” and “perfectly cooked” might rank higher than the “Spicy Shrimp Scampi” for users who prioritize overall positive sentiment over a slightly higher average rating.
Impact of Restaurant Attributes on Ratings and Recommendations
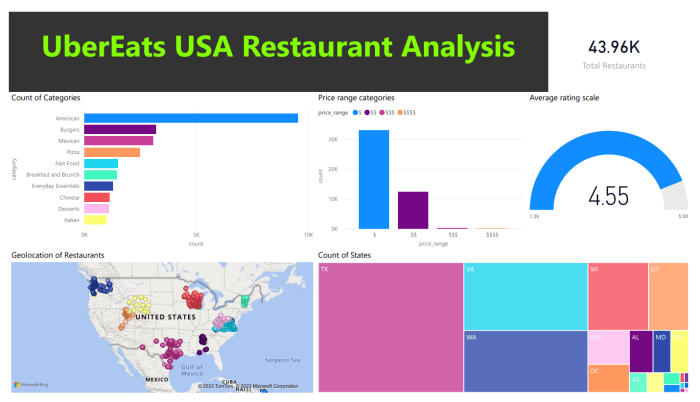
Source: githubusercontent.com
The success of a restaurant on Uber Eats isn’t just about the food itself; it’s a complex interplay of factors influencing customer perception and ultimately, their ratings. Understanding these attributes – from the type of cuisine offered to the speed of delivery – is crucial for both restaurants and users navigating the platform. This section explores the key attributes that significantly impact Uber Eats ratings and how they shape dish recommendations.
Restaurant attributes are powerful predictors of both overall restaurant ratings and individual dish popularity. Certain cuisines tend to garner higher ratings, while price point and delivery speed play a significant role in customer satisfaction. This correlation influences the algorithm’s recommendations, prioritizing restaurants and dishes that consistently receive positive feedback based on these attributes.
Restaurant Attributes and Their Correlation with Uber Eats Ratings
The following table summarizes the relationship between key restaurant attributes and Uber Eats ratings. Note that these correlations are based on general observations and may vary depending on location and specific restaurant performance.
Attribute | Correlation with Rating | Example | Impact on Dish Recommendations |
---|---|---|---|
Cuisine Type | Positive correlation for popular cuisines (e.g., Italian, Mexican, Indian); weaker correlation for niche cuisines. | Highly-rated Italian restaurants tend to receive more orders and higher ratings than restaurants offering less popular cuisines. | Popular cuisine dishes are more likely to be recommended. |
Price Range | Moderate correlation; higher-priced restaurants may receive higher ratings but also face stricter scrutiny. | A high-end sushi restaurant might receive rave reviews but a slightly higher price point might deter some users. | Recommendations balance price and rating; high-rated but expensive dishes might be shown less frequently to budget-conscious users. |
Delivery Speed | Strong positive correlation; faster delivery times lead to higher satisfaction and ratings. | Restaurants consistently delivering within the estimated time window often receive higher ratings than those with frequent delays. | Dishes from restaurants known for fast delivery are more likely to appear in recommendations, particularly for users prioritizing speed. |
Visual Representation of Restaurant Attributes and Dish Popularity
Imagine a three-dimensional scatter plot. The X-axis represents the average delivery time, the Y-axis represents the average price point of dishes, and the Z-axis represents the average dish rating. Each dot represents a dish, with its position determined by its delivery time, price, and rating. Dishes with high ratings would cluster towards the top of the plot, while those with slow delivery times or high prices might be scattered lower, even if their ratings are relatively high. The density of the dots would also indicate popularity; denser clusters represent more popular dishes within specific attribute combinations. This visual would clearly demonstrate how the interplay of delivery speed, price, and rating contributes to a dish’s overall popularity and likelihood of appearing in recommendations.
User Behavior and Rating Patterns
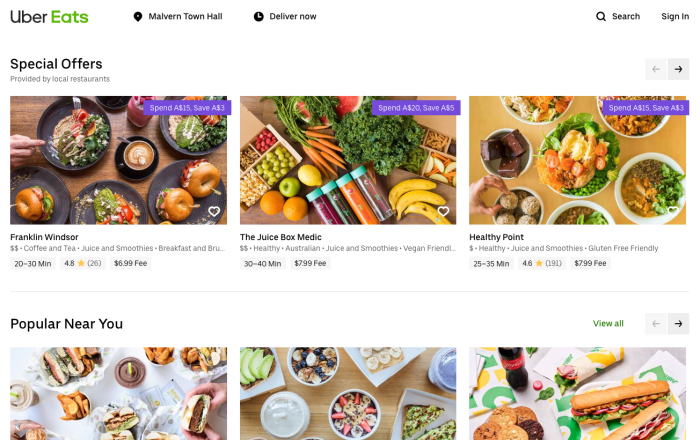
Source: uberinternal.com
Understanding how users interact with Uber Eats and the factors influencing their ratings is crucial for restaurants aiming to optimize their online presence. This involves analyzing review content, identifying rating trends, and considering the diverse demographics of the Uber Eats user base. By dissecting user behavior, we can uncover valuable insights into what drives positive and negative reviews, ultimately helping restaurants improve their offerings and customer satisfaction.
User reviews on Uber Eats offer a rich source of data, revealing patterns and preferences. Analyzing these reviews helps identify areas for improvement and highlights aspects of the dining experience that resonate most with customers. The data allows for a granular understanding of what truly matters to the Uber Eats user – going beyond simple star ratings to understand the nuances of customer feedback.
Categorization of User Reviews
To effectively analyze user reviews, we can categorize them based on recurring themes. This structured approach allows for a more focused understanding of the specific aspects of the dining experience that contribute to user satisfaction or dissatisfaction.
- Taste: This category encompasses comments directly related to the flavor profile of the dish, including descriptions of taste, seasoning, and overall palatability.
- Portion Size: Reviews in this category focus on the quantity of food received, comparing it to expectations and value for the price.
- Presentation: This category considers the visual appeal of the dish, including factors such as plating, arrangement, and overall aesthetic quality upon delivery.
- Packaging: Reviews here focus on the quality of the packaging, its ability to maintain food temperature and prevent spills or damage during delivery.
- Delivery Time and Temperature: This category addresses the speed and efficiency of delivery, as well as the temperature of the food upon arrival.
- Accuracy of Order: This includes comments on whether the order was correct, complete, and matched the description on the app.
- Customer Service: This encompasses reviews related to interactions with restaurant staff, either in-person or via phone/app.
Typical User Behavior When Rating Dishes
Several factors influence a user’s decision when rating a dish on Uber Eats. These factors often interact, leading to a complex interplay of experiences that ultimately shape the rating provided.
Users typically base their ratings on a combination of their expectations, prior experiences with similar restaurants, and their overall satisfaction with the entire ordering process. A delicious dish might receive a lower rating if the delivery was late or the food arrived cold. Conversely, a slightly less-than-perfect dish might receive a higher rating if the delivery was prompt and the customer service was excellent. The overall experience, not just the food itself, heavily influences the final rating.
So, you’re scrolling through Uber Eats, agonizing over restaurant ratings and dish recommendations? It’s a real galaxy far, far away from a simple decision, right? Choosing the perfect meal can feel as epic as deciding which star wars live action tv series to binge-watch next. Ultimately, though, the force (of hunger) is strong with one dish, and only one – so choose wisely!
Rating Patterns Across Demographics
Analyzing rating patterns across different demographics reveals interesting insights into varying preferences and expectations. While generalizations can be risky, certain trends often emerge.
For example, younger demographics (18-35) might place a higher emphasis on trendy dishes and unique presentation, while older demographics (55+) might prioritize familiar cuisine and generous portion sizes. Similarly, location can influence rating patterns, with urban users potentially showing a greater preference for diverse cuisine options and quicker delivery times compared to suburban or rural users. These variations highlight the need for restaurants to tailor their offerings and marketing strategies to specific demographic groups to maximize positive reviews and appeal to a broader customer base. For instance, a restaurant specializing in gourmet burgers might find higher ratings among younger urban populations, while a family-style Italian restaurant might garner better reviews from older suburban families.
Improving the Uber Eats Recommendation System
Uber Eats, like any successful food delivery platform, relies heavily on a robust recommendation system. Currently, while functional, the system can be significantly improved to better cater to individual user preferences and provide more accurate, satisfying recommendations. This involves leveraging user data more effectively, incorporating nuanced feedback, and refining the user interface to highlight key information.
The current recommendation system, while using ratings and order history, often falls short in predicting truly unique or adventurous choices. Improving the system requires a multi-pronged approach focusing on data integration, algorithm refinement, and user experience design.
Enhanced Data Integration for Personalized Recommendations
To personalize recommendations more effectively, Uber Eats should integrate a wider range of user data. This goes beyond simple order history and ratings. Consider incorporating data on dietary restrictions (vegetarian, vegan, gluten-free, etc.), preferred cuisines, price sensitivity, and even delivery time preferences. This richer dataset allows for the creation of highly tailored recommendations, moving beyond simple “you might also like” suggestions based solely on past orders. For example, a user who consistently orders inexpensive Thai food on weekdays but splurges on sushi on weekends would receive different recommendations depending on the day and time of their order. This granular approach increases the likelihood of a successful recommendation.
Utilizing User Feedback for Improved Accuracy
User feedback is crucial for refining the recommendation algorithm. Currently, star ratings are utilized, but this is limited. Implementing a more detailed feedback mechanism, such as allowing users to rate specific aspects of a dish (taste, presentation, portion size), would provide much richer data. This detailed feedback can be weighted and incorporated into the algorithm to improve the accuracy of future recommendations. For instance, if many users rate a specific dish highly for taste but negatively for portion size, the algorithm can adjust recommendations accordingly, suggesting similar dishes with larger portions or suggesting side dishes to complement the smaller portions.
Improved User Interface for Ratings and Recommendations
The current Uber Eats app displays ratings and recommendations in a relatively straightforward manner. However, a redesign could significantly enhance the user experience. Imagine a redesigned interface where restaurant ratings are prominently displayed with a visual representation, such as a star rating system alongside a percentage breakdown of positive reviews. Below the rating, a carousel could showcase top-rated dishes, each with its own individual rating and a high-quality image. This carousel could dynamically update based on the user’s preferences and past orders. Furthermore, the system could offer filtering options based on dietary restrictions, cuisine type, price range, and average preparation time. This enhanced visual presentation and interactive filtering would allow users to easily find restaurants and dishes that perfectly match their needs and preferences. The visual hierarchy should prioritize high-rated dishes and restaurants, and clear visual cues (like color-coding or badges) could be used to highlight popular choices or user-specific recommendations.
Ultimate Conclusion
Mastering the art of choosing the perfect meal on UberEats is no longer a gamble. By understanding the interplay of restaurant ratings, user reviews, and key restaurant attributes, you can confidently navigate the app and consistently discover delicious food. This exploration of UberEats data provides a framework for smarter ordering, ensuring every delivery is a satisfying culinary adventure. So, ditch the guesswork and start making informed choices – your taste buds will thank you.