AI spot art forgeries brushstrokes: Forget your grandma’s magnifying glass – the future of art authentication is here, and it’s powered by artificial intelligence. We’re diving deep into how AI algorithms are analyzing the subtle nuances of brushstrokes to separate the genuine masterpieces from the cleverly crafted fakes. This isn’t just about detecting obvious forgeries; we’re talking about the microscopic details, the almost imperceptible variations that reveal the hand of the artist (or the forger).
From machine learning models trained on vast datasets of authentic artwork to the sophisticated image processing techniques used to dissect brush pressure, direction, and texture, we’ll explore the fascinating world of AI-driven art authentication. We’ll uncover both the remarkable successes and the inherent limitations of this technology, considering its potential impact on the art market and the ethical questions it raises.
AI’s Role in Art Forgery Detection
The art world, with its multi-million dollar masterpieces and a long history of skillful forgeries, is increasingly relying on artificial intelligence to authenticate artworks. AI’s ability to analyze vast amounts of data with incredible speed and precision offers a powerful new tool in the fight against art fraud. This technology is moving beyond simple visual comparisons, delving deep into the subtle nuances of brushstrokes to identify even the most sophisticated fakes.
AI algorithms are revolutionizing art authentication by offering a scientific and objective approach to a previously subjective process. This objective analysis helps experts make more informed decisions, reducing reliance on solely human intuition and potentially biased opinions.
AI Algorithms for Brushstroke Analysis
AI uses various algorithms to analyze brushstrokes, focusing on features invisible to the naked eye. Convolutional Neural Networks (CNNs), for example, are particularly adept at identifying patterns within images. These networks are trained on extensive datasets of authentic artworks, learning to recognize the unique characteristics of different artists’ brushwork. Another approach involves using Recurrent Neural Networks (RNNs), which are well-suited to analyzing the sequential nature of brushstrokes, capturing the temporal flow and rhythm of the artist’s hand. These algorithms identify subtle variations in pressure, direction, and speed that distinguish authentic works from forgeries.
Training Machine Learning Models for Brushstroke Recognition, Ai spot art forgeries brushstrokes
The training of these machine learning models involves feeding them a massive dataset of authenticated artworks. This dataset should include a wide variety of paintings from different artists, periods, and styles to ensure the model learns to generalize effectively. The model learns to associate specific brushstroke features with particular artists, enabling it to differentiate between authentic works and imitations. For instance, a model trained on a large collection of Van Gogh paintings would learn to recognize his characteristic impasto style, the thickness of the paint, and the energetic, swirling strokes. This learned knowledge allows the model to flag deviations from these established patterns as potential forgeries.
Key Brushstroke Features Analyzed by AI
AI systems focus on several key features of brushstrokes to detect forgeries. These include:
- Pressure: The amount of pressure applied by the brush varies throughout the stroke, creating unique variations in line width and paint texture. AI can analyze these pressure variations to identify inconsistencies.
- Direction: The direction of brushstrokes reveals much about the artist’s technique and style. AI can identify unnatural or inconsistent directions that may indicate forgery.
- Texture: The texture of the paint, including its thickness (impasto) or thinness, is a crucial indicator of authenticity. AI can analyze digital images to quantify these textural features.
- Speed: The speed of the brushstroke can leave subtle marks that AI can detect. Forgeries often exhibit a lack of the natural variation in speed seen in authentic works.
- Color blending: The way colors are blended can also be analyzed by AI to spot inconsistencies in forgeries. Authentic brushstrokes often show subtle gradations and layering of colors.
Comparison of AI Techniques for Art Forgery Detection
Different AI techniques offer varying strengths and weaknesses in art forgery detection. CNNs excel at recognizing spatial patterns within brushstrokes, while RNNs are better at capturing the temporal dynamics. Some systems combine multiple AI techniques to leverage their complementary strengths, creating a more robust and accurate detection system. For example, a system might use CNNs to analyze the texture and pressure of brushstrokes and then use RNNs to analyze the sequence and rhythm of the strokes. The choice of technique often depends on the specific characteristics of the artwork and the type of forgery being investigated. The continuous development and refinement of these techniques promise even more accurate and sophisticated forgery detection in the future.
Brushstroke Analysis Techniques
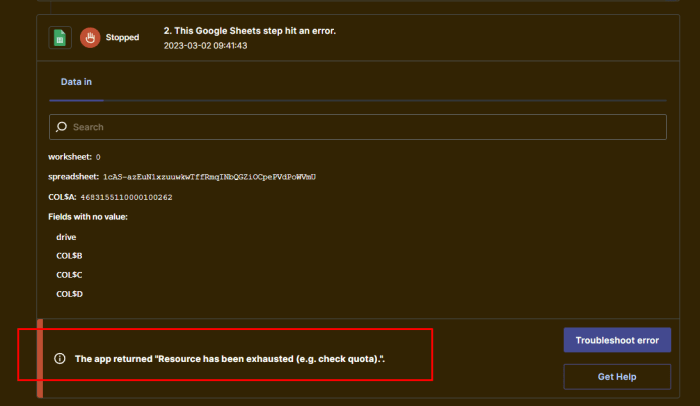
Source: insided.com
Unmasking art forgeries is no longer solely the domain of expert eyes. Sophisticated image processing techniques, coupled with the power of artificial intelligence, are revolutionizing the field of art authentication. By analyzing the subtle nuances of brushstrokes, AI can help distinguish authentic masterpieces from cleverly crafted imitations.
The analysis of brushstrokes relies heavily on image processing techniques that extract quantitative features from digital images of paintings. These techniques go beyond simple visual inspection, delving into the intricate details that define an artist’s unique style. Computer vision plays a crucial role in this process, enabling the identification of subtle variations in brushstroke characteristics that might be imperceptible to the human eye.
Image Processing for Brushstroke Analysis
Image processing begins with high-resolution digital scans of the artwork. Algorithms then enhance the image, focusing on edge detection and texture analysis to isolate the brushstrokes. Techniques like Gabor filters, which are sensitive to orientations and frequencies, are employed to capture the direction, length, and curvature of each stroke. Furthermore, wavelet transforms are used to decompose the image into different frequency components, allowing for the analysis of fine details within the brushstrokes. This multi-faceted approach ensures a comprehensive understanding of the brushstroke characteristics.
Computer Vision and Subtle Brushstroke Variations
Computer vision algorithms are trained on large datasets of authenticated artworks, learning to recognize patterns and variations in brushstrokes associated with specific artists. These algorithms can identify subtle differences in pressure, speed, and direction, as well as variations in paint consistency and texture. For example, an algorithm might detect slight inconsistencies in the spacing between brushstrokes, or variations in the width of individual strokes, that a human eye might miss. This level of detail is crucial in differentiating between the work of a master and a skilled forger.
Quantifying and Classifying Brushstroke Features
AI algorithms can quantify brushstroke features through various metrics. For instance, the average length and width of brushstrokes, their orientation, and the density of strokes within a given area can be measured and statistically analyzed. Machine learning techniques, such as Support Vector Machines (SVMs) or neural networks, are then used to classify these features, creating a unique “brushstroke fingerprint” for each artist. This fingerprint can then be compared to the brushstrokes in a questioned painting to assess authenticity. For example, a statistical analysis might reveal a significant difference in the average brushstroke length between a suspected forgery and authenticated works by the same artist, suggesting a high probability of forgery.
Hypothetical Workflow for AI-Based Brushstroke Analysis
Below is a hypothetical workflow demonstrating how AI could be used to analyze a painting’s brushstrokes. The process involves several steps, from image acquisition to final classification.
Step | Input | Processing Method | Output |
---|---|---|---|
1. Image Acquisition | High-resolution digital image of the painting | Digital scanning | Digital image file (e.g., TIFF) |
2. Preprocessing | Digital image file | Noise reduction, color correction, image enhancement | Cleaned and enhanced digital image |
3. Brushstroke Segmentation | Enhanced digital image | Edge detection, texture analysis (e.g., Gabor filters, wavelet transforms) | Segmented image highlighting individual brushstrokes |
4. Feature Extraction | Segmented image | Measurement of brushstroke length, width, orientation, curvature, density, etc. | Set of quantitative brushstroke features |
5. Classification | Quantitative brushstroke features | Machine learning algorithms (e.g., SVM, neural networks) trained on a database of authenticated artworks | Probability score indicating authenticity/forgery |
Limitations of AI in Spotting Forgeries
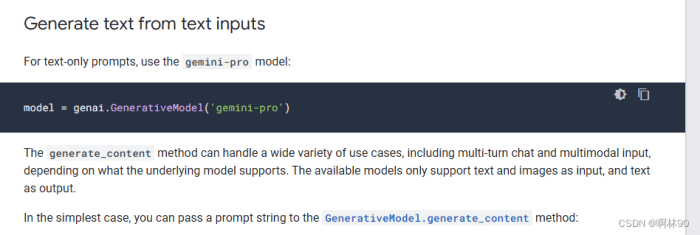
Source: csdnimg.cn
AI’s ability to analyze brushstrokes is getting seriously sophisticated, helping to spot art forgeries with impressive accuracy. This precision reminds me of another clever automated system – check out this article on how email bot waste scammers time by cleverly mimicking human interaction. Just like those bots, AI art forgery detection is all about efficiently identifying patterns and wasting the time of those trying to pull a fast one – only instead of scammers, it’s art fraudsters.
AI is revolutionizing art authentication, but it’s not a magic bullet. While algorithms can analyze brushstrokes and pigment composition with impressive speed and precision, the complexities of art forgery present significant hurdles that even the most advanced AI struggles to overcome. Sophisticated forgeries, crafted by skilled artists employing techniques designed to mimic the masters, often evade detection by current AI systems.
AI-driven forgery detection systems rely on training data, and the quality and diversity of this data directly impact their accuracy. A system trained primarily on a specific artist’s work might struggle to identify forgeries of that artist’s style created by someone familiar with those specific techniques. This inherent limitation highlights the risk of bias in AI-based authentication. Furthermore, the sheer variety of artistic styles, techniques, and materials used throughout history makes creating a truly comprehensive training dataset an almost impossible task. The constant evolution of forgery techniques also renders existing AI models quickly outdated.
AI Bias and Error in Forgery Detection
The accuracy of AI forgery detection is heavily reliant on the quality and representativeness of its training data. If the dataset predominantly features forgeries of a particular style or technique, the AI might become overly sensitive to those specific characteristics, potentially misidentifying authentic works with similar traits as forgeries. Conversely, a dataset lacking sufficient examples of a particular forgery technique could lead to the AI missing those subtle clues. This highlights the inherent risk of bias, a critical limitation that can lead to inaccurate classifications and potentially damaging misattributions. For instance, an AI trained primarily on digital forgeries might misinterpret the subtle texture variations in a traditional oil painting, leading to a false positive.
The Indispensable Role of Human Expertise
Despite the advancements in AI, human expertise remains crucial in art authentication. AI excels at analyzing large datasets and identifying patterns that might escape the human eye, but it lacks the nuanced understanding of artistic context, historical trends, and the subtle nuances of an artist’s evolution that a seasoned expert possesses. A human art historian can consider factors such as provenance, the artist’s biography, and the historical context surrounding the artwork, elements that AI currently struggles to incorporate effectively. Therefore, a collaborative approach, where AI assists human experts by pre-screening works and highlighting potential areas of concern, offers the most promising path towards accurate authentication.
Comparing AI and Traditional Methods
Traditional art authentication methods, which rely heavily on stylistic analysis, material examination, and provenance research, have long been the gold standard. These methods, while time-consuming and subjective, have proven effective in identifying many forgeries. AI-based methods offer the potential for faster and more objective analysis, particularly in identifying subtle inconsistencies in brushstrokes or pigment composition. However, AI’s current limitations mean that it cannot yet replace the comprehensive approach of traditional methods. A combination of both approaches—leveraging AI’s analytical power while retaining human expertise for contextual interpretation—presents the most robust and reliable strategy for art authentication in the face of increasingly sophisticated forgeries. The accuracy of AI-based methods is constantly improving, but it is still far from surpassing the multifaceted assessment performed by experienced art historians and conservators.
Future Developments in AI-Powered Art Authentication
The world of art authentication is poised for a revolution. Current AI methods, while impressive, only scratch the surface of what’s possible. Future advancements promise a level of accuracy and efficiency previously unimaginable, transforming how we verify artistic provenance and combat forgery. Imagine a world where a simple smartphone scan could definitively authenticate a masterpiece. That future is closer than you think.
Future AI systems will likely leverage a combination of techniques far beyond current capabilities. This will involve not only analyzing brushstrokes but also incorporating spectroscopic data, material analysis, and even stylistic nuances learned from vast datasets of authenticated artwork. The integration of these diverse data points will create a far more robust and reliable authentication process.
Advanced AI Capabilities in Art Forgery Detection: A Hypothetical Scenario
Imagine a future scenario: A renowned art gallery receives a painting purportedly by Vincent van Gogh. Instead of relying solely on human experts, the gallery uses a sophisticated AI system. This system, trained on millions of images and spectroscopic analyses of authenticated Van Gogh works, begins its analysis. It meticulously examines the canvas texture, pigment composition, brushstroke patterns, and even the subtle variations in the artist’s application of paint – including the microscopic inconsistencies only detectable through advanced imaging techniques. The AI cross-references this data with its vast database, comparing it against known forgeries and authenticated pieces. Within minutes, the system provides a comprehensive report, not only identifying the painting’s authenticity but also pinpointing specific characteristics that support its conclusion. This hypothetical scenario highlights the potential for AI to significantly reduce the time and cost associated with art authentication, while increasing the accuracy of assessments.
Impact of AI on the Art Market and Art Authentication Process
The impact of AI on the art market will be profound. Increased accuracy in authentication will boost investor confidence, potentially leading to increased market liquidity. The process itself will become faster and more efficient, reducing the reliance on human experts and minimizing the potential for human error or bias. This will also democratize access to authentication services, making them more readily available to a wider range of collectors and institutions. We could see the emergence of new art market players who specialize in AI-driven authentication services, offering faster and more affordable options to traditional methods. This could lead to greater transparency and accountability within the art world, combating the illicit trade of forgeries and helping to protect artists’ legacies.
AI-Driven Database of Brushstroke Characteristics
Future AI systems will play a crucial role in creating comprehensive databases of brushstroke characteristics for different artists. These databases will be far more detailed than anything currently available, encompassing not only visual data but also spectroscopic and material analysis. The AI will be trained to identify subtle variations in brushstrokes, even those imperceptible to the human eye. This would allow for a much more nuanced understanding of an artist’s technique and would significantly improve the accuracy of forgery detection. For example, an AI could analyze the subtle pressure variations in a brushstroke, the directionality of the strokes, and the types of paint used, providing a multi-faceted fingerprint of the artist’s technique. This detailed information would then be used to compare against suspect artworks, aiding in the detection of forgeries.
Ethical Considerations of AI in Art Authentication
The increasing use of AI in art authentication raises several ethical considerations:
- Bias in AI training data: If the AI is trained on a dataset that is not representative of the diversity of artistic styles and techniques, it may produce biased results.
- Data privacy and security: The use of AI requires the collection and analysis of vast amounts of data, raising concerns about the privacy and security of this information.
- Accessibility and equity: The cost of implementing AI-powered authentication systems may create barriers to entry for smaller galleries and individual collectors.
- Over-reliance on technology: There is a risk of over-reliance on AI, potentially leading to a decline in the expertise and skills of human art authenticators.
- Transparency and explainability: It is crucial to ensure that the decision-making processes of AI systems are transparent and explainable, so that their conclusions can be understood and challenged.
Illustrative Examples of AI’s Impact: Ai Spot Art Forgeries Brushstrokes
AI’s application in art forgery detection is moving beyond theoretical possibilities and into practical applications, offering exciting new avenues for art authentication. The ability to analyze brushstrokes with unprecedented precision allows for a level of scrutiny previously unattainable by human experts alone. Let’s delve into some hypothetical scenarios showcasing AI’s potential.
AI’s power in art authentication lies in its capacity to process vast amounts of data and identify subtle patterns invisible to the naked eye. This capability is particularly valuable when analyzing brushstrokes, which are often the key to distinguishing a genuine artwork from a forgery.
AI Successfully Identifying a Forgery
Imagine a purported Van Gogh painting, “Sunflowers in Autumn,” surfaces at auction. The painting appears convincingly authentic at first glance, displaying the characteristic impasto style and vibrant colours associated with the artist. However, subtle discrepancies exist. An AI trained on a vast dataset of verified Van Gogh paintings, focusing on parameters like brushstroke pressure, direction, length, and pigment distribution, is employed. The AI detects inconsistencies. Specifically, the AI identifies that the brushstrokes in the suspect painting are too uniformly consistent in pressure and direction, lacking the characteristic variations and spontaneity observed in genuine Van Gogh works. Furthermore, the AI analyzes the pigment layering, revealing a less nuanced and layered application than is typical of Van Gogh’s technique. The AI flags these deviations, ultimately leading experts to conclude that the painting is a forgery.
Distinguishing a Genuine Painting from a Forgery Using AI
Consider a hypothetical landscape painting by Claude Monet, “Misty Morning on the Seine.” Monet’s style is characterized by short, broken brushstrokes that create a shimmering effect of light and movement. These brushstrokes are typically applied with a loose, almost haphazard quality, yet possess a clear underlying structure. To distinguish a genuine Monet from a forgery, AI could be trained on a large dataset of verified Monet paintings. The AI would analyze aspects like the length, direction, and density of the brushstrokes, comparing them to the statistical distribution of these features in the authentic Monet dataset. Additionally, the AI could analyze the color mixing techniques, looking for the characteristic blending and layering of pigments, often visible in the subtle variations within the brushstrokes themselves. A high-quality forgery might mimic the overall impression of the painting, but AI could detect minute differences in the statistical distribution of brushstroke characteristics, color mixing patterns, and underlying canvas texture, ultimately revealing the forgery.
Visual Differences in Brushstrokes Between a Genuine Painting and a Forgery
Imagine comparing a genuine Rembrandt self-portrait with a skilled forgery. In the genuine Rembrandt, the brushstrokes exhibit a remarkable range of pressure, from delicate highlights to heavily impastoed areas, conveying depth and texture. The direction of the brushstrokes flows organically, reflecting the artist’s hand movements and expressive intent. In contrast, the forgery, while perhaps technically proficient, might show more uniformity in brushstroke pressure and direction. The transitions between light and shadow might appear less nuanced, lacking the subtle gradations of color and texture present in the genuine painting. An AI, analyzing the variance in brushstroke characteristics, could quantify these differences. It might identify a statistically significant lack of variability in the forgery’s brushstrokes, compared to the wide range of pressures and directions found in the genuine Rembrandt. Furthermore, the AI could detect the absence of the characteristic “broken” brushstrokes or subtle layering of pigments typical of Rembrandt’s technique, highlighting the artificiality of the forgery.
Final Review
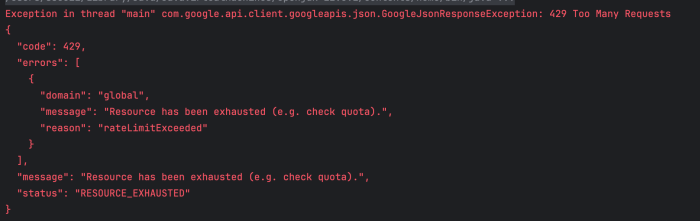
Source: sstatic.net
The ability of AI to analyze brushstrokes and detect art forgeries is rapidly evolving, promising a future where authentication is more precise and efficient. While challenges remain, particularly in identifying highly sophisticated forgeries, the synergy between AI and human expertise is undeniably powerful. The marriage of technological prowess and human intuition is set to reshape the art world, fostering greater transparency and trust in the authenticity of artworks. The brushstrokes may be microscopic, but their significance, amplified by AI, is monumental.