Uber tweaks rating system transparent? That’s the burning question, isn’t it? For years, Uber’s rating system has been a bit of a black box, leaving drivers and riders alike wondering how it actually works and whether it’s truly fair. This deep dive explores the ins and outs of Uber’s rating system, examining its transparency (or lack thereof), its impact on user behavior, and potential improvements that could make the whole process fairer and more understandable for everyone involved. We’ll unpack the complexities, analyze the biases, and ultimately, ask: Can Uber make its rating system truly transparent and equitable?
We’ll delve into the mechanics of the system, looking at how ratings are calculated for both drivers and riders, and how these ratings affect everything from driver earnings to rider access to services. We’ll analyze the potential for bias and explore how Uber could incorporate user feedback more effectively to address concerns about fairness. Prepare for a revealing look behind the curtain of one of the most impactful rating systems in the gig economy.
Uber’s Rating System
Navigating the world of ride-sharing often feels like a delicate dance between driver and rider, a silent negotiation of expectations and experiences. At the heart of this dance lies Uber’s rating system, a seemingly simple yet powerful mechanism shaping both the rider experience and driver livelihood. This system, while seemingly straightforward, has a profound impact on the overall dynamics of the platform.
Uber’s rating system is a two-way street, employing a five-star rating scale for both drivers and riders. Drivers rate riders after each trip, and riders, in turn, rate their drivers. These ratings, accumulated over time, paint a picture of a user’s behavior and contribute to their overall standing within the Uber ecosystem.
Factors Influencing User Ratings
A multitude of factors contribute to a user’s rating. For drivers, these can include punctuality, cleanliness of the vehicle, driving skills (smoothness, adherence to traffic laws), communication (courtesy, helpfulness), and overall professionalism. Riders, on the other hand, are rated on their respect for the driver, cleanliness, punctuality, and clarity of destination. A rider consistently leaving excessive trash in the vehicle or being excessively rude is likely to receive a lower rating. Conversely, a driver who consistently arrives early, drives safely, and engages in pleasant conversation will likely receive higher ratings.
Rating Impact on Earnings and Service Access
Ratings directly influence both driver earnings and rider access to services. Drivers with consistently high ratings often receive priority access to ride requests, leading to more frequent trips and higher earnings. Conversely, drivers with consistently low ratings might face limitations, potentially impacting their income. For riders, very low ratings could lead to temporary or even permanent account suspension, restricting their ability to use the Uber platform. While Uber doesn’t publicly disclose the exact thresholds, maintaining a decent rating is crucial for continued access to the service for both drivers and riders. Think of it like a credit score, but for ride-sharing. A consistently low rating might lead to limited opportunities or even account restrictions.
Comparison of Driver and Rider Rating Systems
Feature | Driver Rating | Rider Rating | Impact |
---|---|---|---|
Rating Scale | 1-5 stars | 1-5 stars | Determines driver income and rider access to service |
Rating Factors | Driving skills, cleanliness, punctuality, communication, professionalism | Respect for driver, cleanliness, punctuality, clear destination | Directly influences the rating given |
Frequency | After each trip by the rider | After each trip by the driver | Consistent ratings build a profile over time |
Consequences of Low Rating | Reduced ride requests, potential income reduction | Limited or suspended access to the app | Significant impact on user experience and platform access |
Transparency in the Uber Rating System
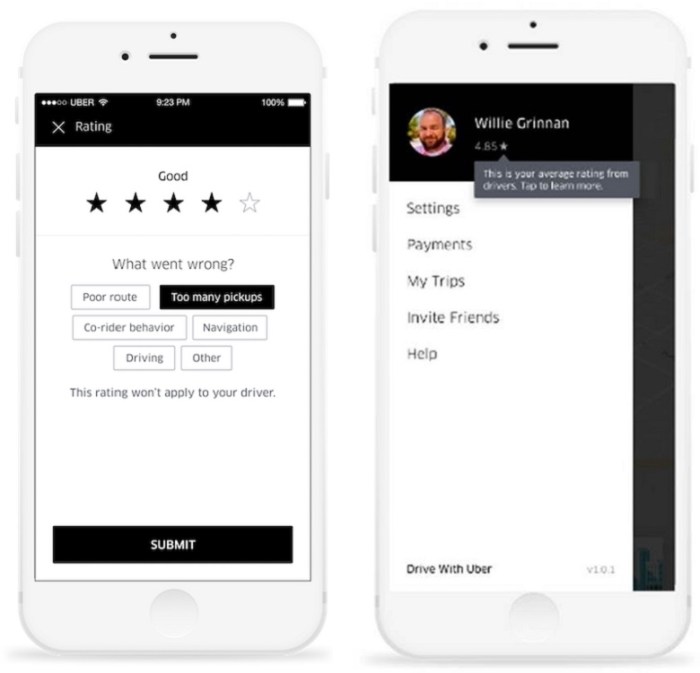
Source: fonearena.com
The Uber rating system, while seemingly straightforward, operates within a veil of limited transparency that impacts both drivers and riders. Understanding the specifics of how ratings are calculated, how they affect driver earnings, and what constitutes a low rating can be surprisingly opaque, leading to frustration and uncertainty for all involved. This lack of clarity creates a system where fairness and accountability can be easily questioned.
Areas Lacking Transparency in the Uber Rating System
Uber’s rating system is a black box for many users. The exact algorithm used to calculate ratings isn’t publicly available, leaving drivers and riders guessing about the factors influencing their scores. For drivers, the lack of detailed feedback on negative ratings makes it difficult to improve performance. Riders, on the other hand, are often unclear on how their ratings impact their ability to access rides or receive promotions. Furthermore, the impact of a low rating on driver income or rider access to services is not explicitly explained. The thresholds for achieving certain statuses (e.g., top-rated driver) remain ambiguous, creating a sense of arbitrariness in the system.
Consequences of Lack of Transparency for Drivers and Riders
The lack of transparency in Uber’s rating system creates several negative consequences. For drivers, a low rating can lead to deactivation, reduced earnings due to fewer ride requests, and a lack of opportunities for bonuses or incentives. The inability to understand why they received a low rating prevents them from addressing the underlying issues. For riders, a low rating might result in longer wait times for rides, difficulty booking during peak hours, or even account suspension. The ambiguity surrounding rating calculations fosters a climate of distrust and uncertainty, damaging the overall user experience.
Improving Transparency in the Uber Rating System
Uber could significantly improve transparency by publicly disclosing the key factors contributing to ratings. This could include a breakdown of the weighting given to factors like on-time arrival, cleanliness of the vehicle, driver behavior, and rider behavior. Providing more detailed feedback to drivers on negative ratings, including specific examples, would empower them to make improvements. Similarly, riders could benefit from clearer explanations of how their ratings affect their experience. Implementing a system that allows drivers to appeal unfair ratings and provides a clear appeals process would further enhance fairness and accountability. Finally, publishing clear metrics and thresholds for achieving different rating levels would increase predictability and reduce the feeling of arbitrariness.
Examples of Transparent Rating Systems in Other Companies
Companies like Airbnb provide much more detailed feedback in their rating system. Hosts receive specific comments from guests, allowing them to identify areas for improvement. Similarly, platforms like Yelp often include detailed reviews that explain the rationale behind ratings. These systems foster a more constructive and transparent environment, benefiting both providers and consumers. These examples demonstrate that more transparency is not only possible but also beneficial for creating a more positive and productive user experience.
Impact of Ratings on Driver and Rider Behavior
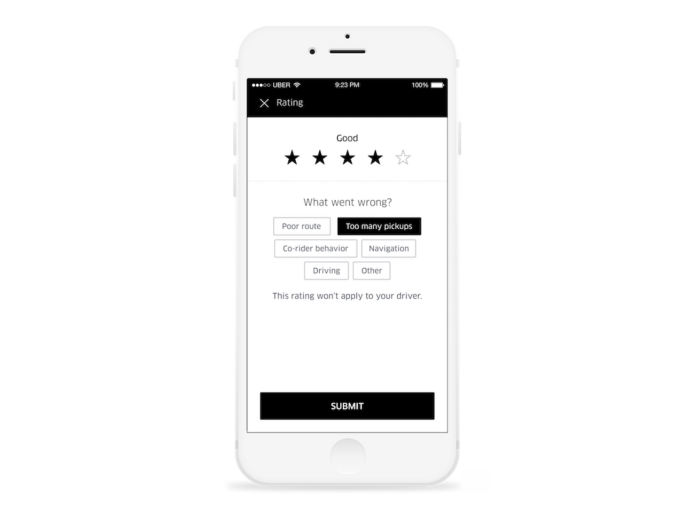
Source: techcrunch.com
The Uber rating system, while seemingly simple, exerts a powerful influence on the behavior of both drivers and riders, shaping their interactions and ultimately, the overall platform experience. This two-way street of ratings creates a dynamic feedback loop, constantly adjusting expectations and performance. Understanding this impact is crucial for optimizing the platform and improving the experience for everyone involved.
The current rating system significantly impacts driver behavior across various aspects of their work. Drivers, aware that their ratings directly affect their income potential and even their continued employment on the platform, often adjust their driving styles and customer service strategies to maintain high ratings.
Driver Behavior Modification
Drivers strive for high ratings, leading to changes in their driving practices. This often translates to smoother, safer driving, with a greater emphasis on following traffic laws and adhering to speed limits. They also become more attentive to passenger comfort, adjusting the temperature, playing appropriate music (or not playing any at all, depending on passenger preference), and offering engaging conversation or maintaining a quiet and respectful atmosphere as per the passenger’s cues. Conversely, drivers with consistently low ratings might experience increased stress and pressure, potentially leading to less careful driving or diminished customer service. The constant pressure to maintain a high rating can lead to a degree of performance anxiety for some drivers.
Rider Behavior Modification
Rider ratings, while less directly impactful on their immediate experience than driver ratings are on drivers, still influence their behavior. Riders who consistently receive low ratings might become more self-conscious about their interactions with drivers, paying closer attention to their behavior and attempting to be more considerate. They might be more likely to communicate their destination clearly, maintain a clean environment in the vehicle, and treat the driver with respect. Conversely, riders with high ratings might feel more empowered to request specific accommodations or express their preferences with more confidence, knowing they have a positive track record.
Comparative Impact on User Experience
The impact of ratings differs significantly between drivers and riders. For drivers, ratings directly affect their livelihood. A low rating can lead to reduced income, deactivation from the platform, and increased stress. For riders, the impact is less direct, primarily influencing their perception of the service and their future interactions. While a low rating might not have immediate financial consequences, it can lead to difficulties in securing rides, especially during peak hours, and a feeling of being unfairly penalized.
Hypothetical Scenario: Increased Transparency, Uber tweaks rating system transparent
Imagine a scenario where Uber implemented a system providing more detailed feedback on ratings. Instead of just a numerical score, drivers receive specific comments from riders explaining the reasons behind their ratings. Similarly, riders receive more nuanced feedback on their behavior from drivers. This increased transparency could lead to several behavioral shifts. Drivers might become more proactive in addressing specific concerns raised by riders, leading to improved service and a higher average rating. Riders, in turn, might become more aware of their impact on the driver experience, leading to greater courtesy and respect. While some drivers might initially feel pressured by more detailed feedback, the overall effect could be a more positive and productive environment for both parties, fostering a more collaborative and understanding atmosphere.
Potential Improvements to the System
Uber’s rating system, while functional, could benefit from some serious upgrades to enhance fairness, transparency, and user experience. A more nuanced approach would foster a more positive and productive environment for both drivers and riders. Let’s explore some key areas for improvement.
Improving the system requires a multi-pronged approach focusing on enhanced transparency, more detailed feedback mechanisms, and fairer rating criteria. This isn’t just about tweaking numbers; it’s about building a system that truly reflects the quality of interactions and promotes responsible behavior.
Increased Transparency in Rating Calculations
The current Uber rating system lacks transparency. Users are given a single numerical score, offering little insight into the factors contributing to that score. Improvements could include providing a breakdown of ratings received over a specific period, categorized by factors like punctuality, cleanliness, communication, and driving skills (for drivers) or politeness, clear directions, and timely pickup (for riders). This granular view allows users to identify specific areas needing improvement, promoting self-reflection and behavioral adjustments. For example, a driver consistently receiving low ratings for cleanliness could focus on maintaining a cleaner vehicle. Similarly, a rider receiving low ratings for communication could work on improving their communication with the driver.
Detailed Feedback Mechanisms for Users
Instead of a simple numerical score, Uber could implement a system providing more comprehensive feedback. This could involve allowing users to leave short, text-based comments alongside their ratings, similar to the review systems used on other platforms like Airbnb or Yelp. Naturally, Uber would need robust moderation systems to prevent abusive or inappropriate comments, but the added context could be invaluable. For example, a driver might receive a low rating with a comment explaining that the rider felt the driver was unnecessarily aggressive in traffic. This would provide context and allows the driver to understand the issue from the rider’s perspective. This could be further enhanced by allowing drivers to respond to comments, fostering open communication and potentially resolving misunderstandings.
Features Enhancing Fairness and Accuracy
Several features could improve the fairness and accuracy of the Uber rating system. One crucial element is introducing a mechanism to flag or filter out potentially biased or unfair ratings. This could involve algorithms identifying unusual rating patterns or allowing users to report suspicious activity. Another important improvement would be to implement a system that weighs ratings differently based on factors such as the frequency of rides or the length of the trip. A single bad rating from a short trip shouldn’t carry the same weight as multiple consistent negative ratings from longer trips. Additionally, implementing a system that allows for rating appeals or dispute resolution could further enhance fairness.
Benefits for Drivers and Riders
An improved rating system benefits both drivers and riders. For drivers, increased transparency and detailed feedback provide valuable insights into their performance, enabling them to improve their services and increase their ratings. This, in turn, can lead to more ride requests and higher earnings. For riders, a fairer and more transparent system ensures they receive reliable and high-quality service. The ability to provide more detailed feedback gives riders more control over their experience and contributes to a better overall platform experience. Ultimately, a more refined rating system strengthens the entire Uber ecosystem by fostering accountability and promoting positive interactions.
Addressing Bias and Fairness
The Uber rating system, while seemingly straightforward, presents a complex landscape when considering fairness and bias. A seemingly simple star rating can reflect much more than just a ride’s quality; it can inadvertently amplify existing societal biases, leading to unfair treatment for both drivers and riders. Understanding these biases is crucial to ensuring a truly equitable platform.
Potential Sources of Bias in the Uber Rating System
Bias in the Uber rating system can stem from various sources. One significant factor is implicit bias – unconscious prejudices that influence our judgments. For instance, riders might subconsciously rate drivers of certain ethnicities or genders lower, even if the ride itself was perfectly acceptable. Similarly, drivers might rate riders based on assumptions about their socioeconomic status or perceived behavior. Another source is algorithmic bias, where the rating system itself might inadvertently disadvantage certain groups. For example, if the system disproportionately flags drivers from specific areas for low ratings due to higher incident rates in those areas (factors unrelated to driver performance), it creates a biased outcome. Finally, reporting mechanisms can also introduce bias; a disproportionate number of reports against drivers from particular backgrounds might skew ratings even if the reports are not always accurate.
Ethical Implications of Biased Rating Systems
Biased rating systems have significant ethical implications. Unfair ratings can lead to job insecurity for drivers, potentially impacting their income and livelihood. For example, consistently lower ratings might result in deactivation from the platform, causing financial hardship. This disproportionately affects marginalized groups who might already face systemic disadvantages. Furthermore, biased ratings can perpetuate harmful stereotypes and reinforce existing inequalities. A system that systematically disadvantages certain groups erodes trust and undermines the platform’s commitment to fairness and inclusivity. Ultimately, a biased system fails to provide a level playing field for all participants, contravening the principle of equal opportunity.
Solutions to Mitigate Bias in the Rating System
Addressing bias requires a multi-pronged approach. Uber could implement blind rating systems, removing identifying information like driver photos or rider profiles during the rating process. This helps reduce the influence of implicit biases. They could also develop more robust algorithms that account for contextual factors and prevent disproportionate flagging of certain groups. For example, the algorithm could consider the historical average rating of a driver in relation to the area they operate in. Regular audits of the rating system to identify and address any emerging biases are crucial. Transparency about the rating system’s workings and the data it uses is also essential to build trust and identify potential areas for improvement. Finally, comprehensive driver and rider education on bias awareness could encourage more conscious and equitable rating practices.
Strategies for Ensuring Fairness in the Rating Process
The following strategies are essential to ensure fairness in Uber’s rating system:
- Implement a more nuanced rating system that allows for detailed feedback beyond a simple star rating, allowing for context and specificity.
- Develop clear guidelines for reporting and handle complaints with impartiality and thorough investigation.
- Regularly audit the system for bias, using statistical analysis to identify any disparities.
- Provide opportunities for drivers and riders to appeal unfair ratings.
- Invest in training and education programs to promote bias awareness among both drivers and riders.
- Increase transparency regarding the rating algorithm and its workings.
The Role of User Reviews and Feedback
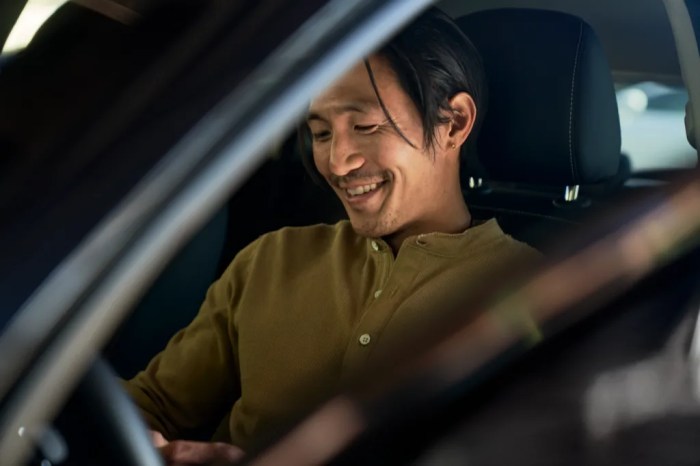
Source: uber-cdn.com
User reviews and feedback form the backbone of any successful rating system, and Uber’s is no exception. While the star rating provides a quick snapshot of a ride, the accompanying text reviews offer crucial qualitative data that can illuminate the nuances of the experience, revealing issues beyond a simple numerical score. These reviews offer a rich source of information for both Uber and its users, impacting everything from driver performance evaluations to service improvements.
User feedback currently plays a significant role in Uber’s rating system, influencing driver and rider ratings. Positive reviews boost ratings, contributing to a positive feedback loop where highly-rated drivers attract more riders and vice-versa. Conversely, negative reviews can lead to deactivation for consistent offenders, though the process involves more than just a single negative review. Uber’s algorithms consider a multitude of factors, including the frequency and severity of negative feedback, before taking action. However, the current system’s effectiveness hinges on how effectively Uber leverages this feedback for improvement.
Improved Incorporation of User Feedback
Uber could significantly enhance its use of user feedback by implementing a more sophisticated system for analyzing and categorizing reviews. Currently, the system may struggle to differentiate between genuine complaints and those stemming from misunderstandings or unrealistic expectations. Natural language processing (NLP) techniques could be employed to automatically identify recurring themes and issues within reviews, allowing Uber to address systemic problems more proactively. For example, if a large number of reviews mention consistently late arrivals in a specific area, Uber could investigate logistical issues like traffic patterns or driver allocation in that region. This data-driven approach would move beyond reactive responses to negative feedback and enable preventative measures.
Challenges of Managing and Responding to Negative Feedback
Managing and responding to negative feedback presents several significant challenges. The sheer volume of reviews generated daily requires efficient automated tools and human oversight to identify critical issues. Furthermore, dealing with abusive or false reviews requires careful consideration to ensure fairness and protect both drivers and riders from unfair accusations. Another challenge lies in effectively addressing the emotional aspects of negative feedback. A simple automated response often falls short; a personalized and empathetic response, even if it can’t resolve the issue completely, can significantly improve user satisfaction and demonstrate Uber’s commitment to addressing concerns. This necessitates a robust system for flagging and escalating critical reviews for human review and response.
Effective User Feedback Handling on Other Platforms
Several other platforms offer valuable examples of effective feedback management. Airbnb, for instance, allows hosts to respond to reviews, fostering a dialogue and demonstrating a commitment to addressing concerns. This encourages a more constructive interaction between users. Similarly, Amazon’s detailed review system, with its ability to filter and sort reviews, provides valuable insights to both consumers and vendors. Their system also allows for product improvements based on collective feedback. These platforms highlight the importance of not just collecting feedback, but actively engaging with it and using it to drive improvements and build trust.
Visual Representation of Rating Data
Understanding the distribution of driver and rider ratings is crucial for Uber to ensure fairness and identify areas for improvement. A clear visual representation of this data can significantly enhance transparency and allow both drivers and riders to better understand their position within the rating system.
A compelling visualization would be an interactive histogram displaying the frequency distribution of both driver and rider ratings.
Driver and Rider Rating Distribution Histogram
The horizontal axis of the histogram would represent the rating score, ranging from 1 to 5 stars (or whatever the rating scale is). Each bar would represent a specific rating score (e.g., a bar for 1-star ratings, a bar for 2-star ratings, and so on). The vertical axis would represent the frequency or percentage of ratings received at each score. Two separate histograms, one for drivers and one for riders, would be displayed side-by-side for easy comparison. Each bar could be color-coded (e.g., green for high ratings, yellow for medium ratings, and red for low ratings) to further enhance readability. Additionally, the average rating for both drivers and riders could be displayed as a distinct marker on each histogram. This visual representation would immediately show the overall distribution of ratings, highlighting the concentration of ratings around the average and the frequency of extreme ratings (both high and low). For example, a high concentration of ratings around 4-5 stars for drivers would indicate a generally positive experience for riders. Conversely, a significant number of 1-2 star ratings for drivers would highlight areas requiring attention and potential improvements.
Enhanced Transparency Through Visualization
This interactive histogram would significantly enhance transparency by providing a clear and easily understandable picture of the rating distribution. Both drivers and riders could see where their rating falls within the overall distribution, allowing for better self-assessment and understanding of their performance or behavior. The side-by-side comparison of driver and rider ratings would also highlight any potential imbalances or discrepancies in the rating system. The interactive nature of the histogram, allowing users to hover over bars to see exact numbers and percentages, would further improve data accessibility and comprehension.
Insights Gained from the Visualization
This visual representation offers several key insights. Firstly, it would clearly identify the average rating for both drivers and riders, providing a benchmark for performance. Secondly, it would reveal the spread or variability of ratings, indicating the consistency of experiences. A wide spread might suggest inconsistencies in service quality or user behavior. Thirdly, the visualization could help identify outliers – drivers or riders with exceptionally high or low ratings – allowing for further investigation into potential causes. Finally, it could help Uber identify trends over time, showing how ratings are changing and allowing for proactive adjustments to the system or policies as needed. For instance, a sudden drop in average driver ratings could signal a need to address a specific issue affecting driver performance or rider behavior.
Closure: Uber Tweaks Rating System Transparent
Ultimately, the transparency of Uber’s rating system is crucial for maintaining trust and fairness within its vast network of drivers and riders. While the current system has its shortcomings, there’s a clear path towards improvement. By implementing the suggestions discussed – from providing more detailed feedback to actively mitigating bias – Uber can create a system that is not only more transparent but also more equitable and beneficial for everyone involved. The journey towards a fairer, more transparent rating system is ongoing, and its success hinges on Uber’s commitment to listening to its users and acting on their feedback.