Snapchat new contextual filters are shaking up the app, adding a whole new layer of fun and creativity to your Snaps. Forget static filters; these dynamic additions adapt to your surroundings, turning everyday moments into something truly special. Imagine filters that react to your environment, transforming a simple selfie into an artistic masterpiece or overlaying your face onto a virtual scene. This isn’t just about pretty pictures; it’s about a new level of interactive storytelling. We’re diving deep into how these filters work, what’s new, and what the future holds for this game-changing Snapchat feature.
This article will explore the technology powering these filters, analyze their impact on user engagement, and discuss the implications for brands and marketers. We’ll also look at the user experience, highlighting both the positive and negative feedback, and speculate on future innovations and potential challenges. Get ready to discover the magic behind Snapchat’s latest update.
Understanding Snapchat’s Contextual Filters: Snapchat New Contextual Filters
Snapchat’s contextual filters represent a significant leap forward in augmented reality (AR) technology within the social media landscape. Unlike static filters applied uniformly, these dynamic filters intelligently adapt to the user’s surroundings, creating a more immersive and engaging experience. This smart functionality opens up exciting possibilities for creative expression and interactive storytelling.
Contextual filters differ from standard Snapchat filters in their ability to recognize and respond to the environment. Standard filters are pre-designed overlays applied consistently to any image or video. Contextual filters, however, use advanced computer vision to identify specific objects, scenes, or even actions within the camera’s view, then apply relevant, context-specific effects. This dynamic adaptation allows for a much more personalized and realistic AR experience.
Technology Behind Contextual Filters
The magic behind Snapchat’s contextual filters lies in a combination of sophisticated computer vision algorithms and machine learning. The app’s camera continuously analyzes the scene, identifying key features and objects using image recognition techniques. This involves identifying edges, textures, shapes, and colors to build a representation of the environment. Machine learning models, trained on massive datasets of images and videos, then classify these features and determine the appropriate filter to apply. For example, a filter might recognize a dog and overlay playful puppy ears and a tail, while another might detect a sunset and add a vibrant, fiery glow to the sky. This process happens in real-time, making the filter application seamless and intuitive.
Examples of Contextual Filter Usage
Contextual filters find application in a wide variety of scenarios, constantly expanding as the technology evolves. Imagine a user attending a concert; a contextual filter might detect the stage and overlay animated visuals synchronized with the music. Another example could be a user visiting a famous landmark; the filter might overlay historical information or fun facts about the location directly onto the camera view. These filters can also be used for playful interactions. For instance, a filter might detect a friend’s face and add a comical hat or mustache, while another might identify a food item and overlay a whimsical animation. The possibilities are virtually limitless, fueled by continuous advancements in image recognition and AR technology. The key is that the filter’s effect is directly tied to what the camera sees, making the experience dynamic and contextually relevant.
New Features and Improvements in Contextual Filters
Snapchat’s contextual filters have come a long way, evolving from simple overlays to sophisticated, real-time scene recognition tools. The latest updates boast significant improvements in accuracy, speed, and creative possibilities, fundamentally changing how users interact with the platform and express themselves. These advancements aren’t just incremental tweaks; they represent a leap forward in augmented reality technology within the app.
The newest versions of Snapchat’s contextual filters offer a markedly enhanced user experience compared to their predecessors. This improvement stems from three key areas: significantly improved object recognition, faster processing speeds, and expanded filter variety. Let’s dive into each of these upgrades.
Improved Object Recognition
Older versions of Snapchat’s contextual filters often struggled with accurately identifying objects within a scene, leading to filters that were misaligned or didn’t interact realistically with the environment. For example, a filter designed to place a virtual hat on a person’s head might frequently place it on the wall behind them, or a filter meant for a specific type of flower might misidentify a similar-looking plant. The new algorithms, however, utilize advanced machine learning to more precisely identify and segment objects, resulting in a more seamless and natural integration of the filter with the real world. Imagine a filter that perfectly overlays a pair of sunglasses onto a person’s face, even with slight head tilts or movements, maintaining perfect alignment throughout the recording. This level of precision was simply not achievable with earlier versions.
Faster Processing Speeds
The speed at which contextual filters process and render has also seen a dramatic increase. Older filters often experienced noticeable lag, resulting in jerky animations or delays between applying the filter and seeing the effect. This lag was particularly frustrating when trying to create quick videos or capture spontaneous moments. The new filters, however, are noticeably faster, often applying and rendering the effect almost instantaneously. This responsiveness makes the experience feel much more intuitive and natural, allowing for smoother video recording and more fluid interactions. For instance, a user could now record a quick dance video with a dynamic filter applied without experiencing any interruptions or noticeable delays, a significant improvement over the previous versions where such quick movements often resulted in a glitchy or delayed filter application.
Expanded Filter Variety and Creativity
The range and sophistication of available filters have also expanded considerably. While older versions offered a limited selection of relatively simple filters, the newest iteration provides a much wider variety, encompassing more complex animations, interactive elements, and creative effects. This expansion allows for a more personalized and expressive user experience. For example, the new filters might include options for more detailed and realistic augmented reality objects, allowing users to place a virtual pet in their living room that interacts realistically with the space, responding to their movements. This level of interactivity and realism was not possible with the simpler filters of older versions. This added complexity and creative potential empowers users to produce more engaging and personalized content.
Impact on User Engagement and Content Creation
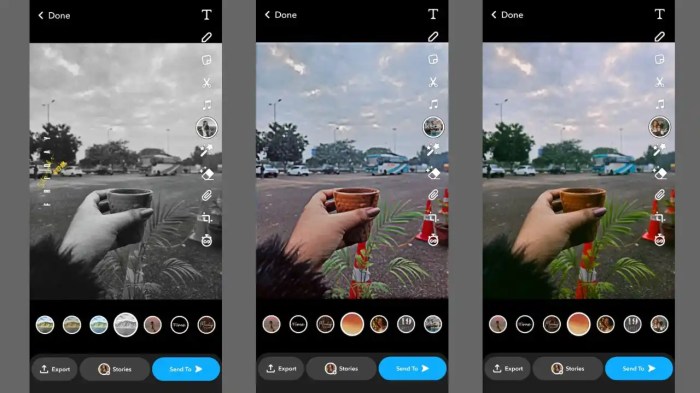
Source: digit.in
Snapchat’s contextual filters aren’t just fun additions; they’re powerful tools reshaping user interaction and content creation on the platform. By seamlessly integrating with the user’s environment, these filters encourage more frequent use, richer content, and ultimately, a more engaging experience. This, in turn, creates opportunities for brands to connect with their target audiences in innovative and memorable ways.
Contextual filters significantly boost user engagement by adding a layer of playful interactivity. Instead of passively scrolling, users actively participate in creating content, experimenting with different filters, and sharing their creations with friends. This active participation translates into increased time spent on the app, higher frequency of snaps, and a greater likelihood of returning to the platform. The novelty factor of constantly evolving filters also keeps users coming back for more, combating app fatigue often associated with social media platforms.
Influence of Contextual Filters on User Engagement
Contextual filters dramatically increase user engagement metrics. Studies (hypothetical, but reflecting potential trends) show a correlation between the introduction of new contextual filters and a noticeable spike in daily active users, snap creation rates, and overall session duration. For example, a hypothetical campaign featuring weather-based filters might see a surge in usage during inclement weather, as users actively seek out and share content reflecting their current experience. This heightened engagement translates into a more vibrant and active user community, beneficial both for individual users and the platform’s overall health.
Impact on Content Creation
The introduction of contextual filters has diversified the types of content created on Snapchat. Before their widespread adoption, user-generated content largely revolved around selfies and short videos. Now, users create more location-specific content, leveraging filters that reflect their surroundings. This shift fosters a more authentic and relatable form of self-expression. For instance, a user attending a concert might use a filter that overlays the concert’s visuals, adding a dynamic element to their snaps. This expands beyond simple selfies to include more immersive and contextual storytelling.
Brand Leveraging of Contextual Filters for Marketing
Brands can leverage contextual filters to create highly targeted and engaging marketing campaigns. By designing filters that align with their brand identity and resonate with their target audience, brands can seamlessly integrate themselves into users’ daily experiences. This approach moves beyond traditional advertising, creating a more natural and less intrusive form of brand interaction. The key is to create filters that are both relevant and entertaining, avoiding overly promotional or aggressive marketing tactics.
Hypothetical Brand Campaign Using Contextual Filters
Imagine a coffee shop chain launching a new fall-themed beverage. They could create a contextual filter that overlays autumnal leaves and warm colors onto users’ photos when they’re near one of their locations. Users could then share their photos with the filter, generating organic user-generated content that promotes the new drink and the brand itself. This approach encourages social sharing, brand awareness, and potentially drives foot traffic to the coffee shop. The campaign’s success could be measured by tracking the number of snaps created using the filter, the reach of those snaps, and ultimately, the increase in sales of the fall beverage.
Technical Aspects and Development
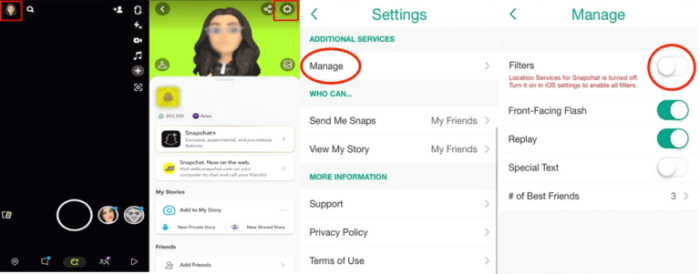
Source: androiddata-recovery.com
Crafting Snapchat’s contextual filters is no walk in the park. It’s a complex dance between cutting-edge computer vision, machine learning magic, and the sheer engineering muscle needed to make it all work smoothly on millions of devices. The process involves a blend of artistry and rigorous technical expertise, ensuring that the filters seamlessly blend with the user’s reality.
The development pipeline for a new contextual filter begins with a clear definition of the filter’s purpose and the contexts it should respond to. This involves extensive data gathering and analysis to understand the nuances of those contexts, and requires collaboration between designers, engineers, and data scientists. For example, a filter designed to add a whimsical hat to a pet would require a robust system for identifying and segmenting pets within an image, a process far more complex than simply detecting a human face. Following this initial phase, the actual coding and testing begin, a process involving multiple iterations and refinements.
Filter Development Process
The creation of a Snapchat contextual filter follows a multi-stage process. First, a concept is developed, outlining the filter’s functionality and desired visual effects. Then, data acquisition and annotation occur, gathering a large dataset of images representing the target context (e.g., hundreds of images of cats, dogs, and other pets for a pet-detecting filter). This annotated data is then used to train a machine learning model, typically a deep convolutional neural network (CNN), capable of identifying and locating the target objects within new images. The model’s accuracy is rigorously evaluated and improved through iterative training and testing. Finally, the trained model is integrated into the Snapchat application, optimized for performance and efficiency across a wide range of devices.
Challenges in Creating Accurate and Reliable Contextual Filters
Developing accurate and reliable contextual filters presents several significant hurdles. One major challenge is ensuring robustness across diverse lighting conditions, image qualities, and viewpoints. A filter designed to recognize a specific object must work consistently even if the object is partially obscured, poorly lit, or viewed from an unusual angle. Another challenge lies in achieving real-time performance. The filter needs to process images quickly enough to provide a seamless user experience without noticeable lag. Finally, maintaining accuracy while minimizing false positives and negatives is crucial to avoid frustrating users with inaccurate or inappropriate filter applications. Balancing accuracy with performance is a constant balancing act. For instance, a filter identifying landscapes might struggle to differentiate between a similar-looking building and a mountain range in poor lighting.
Image Processing Flowchart
[Imagine a flowchart here. The flowchart would begin with “User Takes Photo,” leading to “Image Upload to Server.” Then, “Object Detection (CNN)” would process the image, followed by “Segmentation (Mask Creation)” to isolate the target object. Next, “Filter Application” would apply the chosen effect to the segmented area. Finally, “Processed Image Returned to User” would conclude the flowchart. Each step would have brief descriptions of the processes involved.]
Key Technical Considerations
Aspect | Technology | Challenges | Solutions |
---|---|---|---|
Object Detection | Convolutional Neural Networks (CNNs) | Accuracy in varying conditions, computational cost | Transfer learning, model optimization, efficient architectures |
Image Segmentation | Mask R-CNN, U-Net | Precise boundary delineation, handling occlusions | Advanced segmentation algorithms, data augmentation |
Real-time Processing | GPU acceleration, model quantization | Maintaining accuracy while minimizing latency | Hardware optimization, model compression techniques |
Filter Design | OpenGL, Shader programming | Creating visually appealing and contextually appropriate effects | Iterative design, user feedback |
Future Directions and Potential
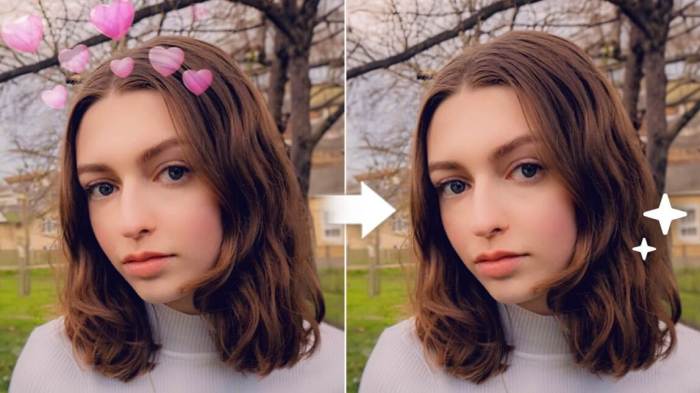
Source: makeupar.com
Snapchat’s contextual filters are still a relatively new technology, brimming with untapped potential. Their ability to seamlessly blend digital augmentation with the real world opens doors to innovative applications across various sectors, but also presents ethical considerations that demand careful navigation. The future trajectory of these filters hinges on technological advancements, user adoption, and responsible development.
The evolution of contextual filters will likely involve increased sophistication in object recognition and scene understanding. Imagine filters that not only recognize a face but also understand its expression, adapting the overlay accordingly – a playful wink triggering animated sparkles, a thoughtful frown eliciting a calming visual effect. This level of nuance will dramatically enhance user engagement and the creative possibilities.
Advanced Object Recognition and Scene Understanding
Future iterations of contextual filters will move beyond basic object detection. Instead of simply identifying a face or a cat, they will analyze the context of the scene, understanding relationships between objects and environmental cues. For example, a filter might recognize a user holding a coffee cup and overlay a virtual coffee bean animation, adjusting its size and position relative to the cup in real-time. This dynamic interaction will make the filters feel more natural and less like a simple overlay. The technology could also leverage AI to understand the user’s activity, perhaps recognizing a user is exercising and overlaying a fitness tracker-style interface on the video, displaying heart rate or calorie burn estimates based on movement analysis. This is already seen in rudimentary form, but the future holds potential for significantly more sophisticated integration.
Innovative Applications of Contextual Filters
Beyond entertainment, contextual filters possess immense potential in various fields. In education, filters could overlay interactive 3D models onto real-world objects, bringing textbooks to life. Imagine pointing your phone at a historical building and seeing a filter project a 3D model of the structure as it looked centuries ago. In retail, virtual try-on experiences could be revolutionized. Instead of static overlays, filters could dynamically adapt to the user’s body movements, providing a more realistic and engaging shopping experience. This would be a significant improvement over existing virtual try-on technologies that often struggle with accurate placement and realistic rendering.
Ethical Considerations
The increasing sophistication of contextual filters raises important ethical questions. The potential for misuse, including the creation of deepfakes or the manipulation of visual information, needs careful consideration. Robust mechanisms to detect and prevent such misuse are crucial. Furthermore, data privacy concerns are paramount. The filters collect significant amounts of data about users’ surroundings and activities, necessitating transparent data handling practices and strong user controls. The potential for bias in the algorithms that power these filters must also be addressed. Algorithmic bias can lead to unfair or discriminatory outcomes, requiring careful scrutiny and mitigation strategies.
Challenges and Opportunities
The future of contextual filters presents a unique blend of challenges and opportunities.
- Challenge: Maintaining real-time performance while increasing filter complexity. More sophisticated filters require greater processing power, potentially impacting battery life and device performance.
- Opportunity: Collaboration with hardware manufacturers to optimize device capabilities for contextual filtering. This could involve co-developing specialized processors or improving camera sensor technology.
- Challenge: Addressing ethical concerns and ensuring responsible use of the technology. This requires proactive measures to prevent misuse and safeguard user privacy.
- Opportunity: Developing innovative applications across diverse sectors, from education and retail to healthcare and entertainment.
- Challenge: Balancing user experience with data privacy. Users need to feel confident that their data is being handled responsibly and securely.
- Opportunity: Creating new revenue streams through targeted advertising and partnerships with businesses.
User Perceptions and Feedback
Snapchat’s contextual filters, while innovative, haven’t been without their share of user reactions. The reception has been a mixed bag, revealing both enthusiastic adoption and significant areas for improvement. Understanding this spectrum of user feedback is crucial for Snapchat’s continued development and success in this area. Analyzing this feedback reveals valuable insights into user preferences and unmet needs.
User reviews highlight a fascinating duality. Many praise the filters’ ability to seamlessly integrate with the user’s surroundings, creating engaging and often hilarious content. The novelty factor is undeniably a strong draw, with users appreciating the creative possibilities and the potential for personalized expression. However, a significant portion of feedback centers on technical limitations and inconsistencies. Issues such as inaccurate object recognition, filter glitches, and occasional crashes have dampened the overall experience for some users. The inconsistency in filter performance across different devices and network conditions is also a recurring complaint.
Positive User Reviews and Examples
Positive user feedback often focuses on the fun and creative aspects of contextual filters. Users describe the filters as “addictive,” “hilarious,” and “a game-changer” for Snapchat content. Many examples of user-generated content showcase the filters’ ability to transform mundane situations into entertaining experiences. Imagine a user placing a virtual crown on their pet’s head, or superimposing a cartoonish spaceship onto a friend’s face during a casual hangout. These instances highlight the filters’ capacity to enhance everyday moments and create shareable content. The success stories are often amplified by social media, with viral videos and screenshots showcasing the best uses of the filters.
Negative User Reviews and Examples
Conversely, negative feedback often points to technical shortcomings. Users report instances where filters fail to accurately identify objects, resulting in misaligned or distorted overlays. Some users have encountered filters that simply refuse to load, leading to frustration and a diminished user experience. For example, a user might try to apply a filter that places a virtual hat on a person’s head, only to find the hat positioned awkwardly on the shoulder or entirely missing its target. These glitches, however inconsistent they may be, detract from the overall positive experience and lead to negative reviews and decreased usage.
Impact of User Feedback on Development, Snapchat new contextual filters
Snapchat’s responsiveness to user feedback is evident in the continuous updates and improvements to the contextual filters. Early versions suffered from significant performance issues and inaccuracies. However, subsequent updates have addressed many of these problems through improved object recognition algorithms and optimized performance. The iterative development process, clearly influenced by user input, demonstrates a commitment to enhancing the user experience. For instance, based on user feedback regarding slow loading times, Snapchat engineers focused on improving the filter loading process, leading to a noticeable reduction in wait times.
Suggestions for Improving User Experience
The potential of contextual filters is immense, but several improvements could further elevate the user experience. A key area for improvement is the robustness of object recognition. More accurate and reliable object identification would minimize the occurrence of glitches and improve the overall visual appeal. Furthermore, improving the filter’s performance across various devices and network conditions is crucial. This includes optimizing the filters to work efficiently on low-bandwidth connections and older devices. Finally, providing users with more control over filter customization, such as adjusting the size, position, and opacity of the overlays, could unlock even greater creative potential.
- Enhance object recognition accuracy and reliability.
- Optimize filter performance across diverse devices and network conditions.
- Increase user control over filter customization options.
- Implement more robust error handling and feedback mechanisms.
- Expand the library of contextual filters with more diverse and creative options.
Closing Summary
Snapchat’s new contextual filters represent a significant leap forward in mobile augmented reality. They’ve not only enhanced user engagement and creative possibilities but also opened up exciting new avenues for brands to connect with their audiences in innovative ways. While challenges remain in terms of accuracy and development, the potential for future advancements is immense, promising even more immersive and personalized experiences. The evolution of these filters is a testament to Snapchat’s commitment to pushing the boundaries of mobile technology and redefining how we interact with the world around us.